By looking outside the current wave of hype, we can create a framework for weighing up the practical impact of AI on any business.
- Large Language Models (LLMs) are just one type of AI. Despite the hype, they have not superseded all other types and made them redundant.
- Many of the most promising applications of AI are emerging in areas of machine learning that have little to do with LLMs.
- We can consider the trajectory AI by thinking about the categories of AI capability: Narrow AI, Artificial General Intelligence, and Super AI.
Adapted with permission from TRANSCEND by Faisal Hoque (Post Hill Press, Hardcover, April 8, 2025). Copyright 2025, Faisal Hoque, All rights reserved.
The current wave of AI hype was kicked off by the rollout and subsequent development of ChatGPT. And in the public mind, it is with this kind of application that AI is most strongly associated — the LLM-powered chatbots that we can talk and listen to in natural language. But it is important to understand that LLMs are just one type of AI. Despite the hype, they have not superseded all other types and made them redundant. Far from it. Many of the most promising applications of AI are emerging in areas of machine learning that have little to do with LLMs. It is important to remember this if we are to make the most of all of AI and guard against all its potential dangers.
The promise of AI extends far beyond the kinds of outputs we get from LLMs. To take just three examples, it also holds the promise of delivering better patient diagnostics and improved drugs with which to treat those patients, improving weather forecasts, and providing more efficient and environmentally friendly methods for utilizing energy. All of these forms of output may turn out to be significantly more important than a chatbot’s ability to create a thousand generic LinkedIn posts. Another enormously significant application of AI that has nothing to do with natural language is in the field of robotics. AI can be — and already is being — used to help with the development of advanced prosthetics. It is already being used to open restaurants and food-trucks where robots cook all the food. It is already being used in hospitals to assist with, or even conduct, surgeries. And again, none of these applications have anything to do with processing and generating natural language text.
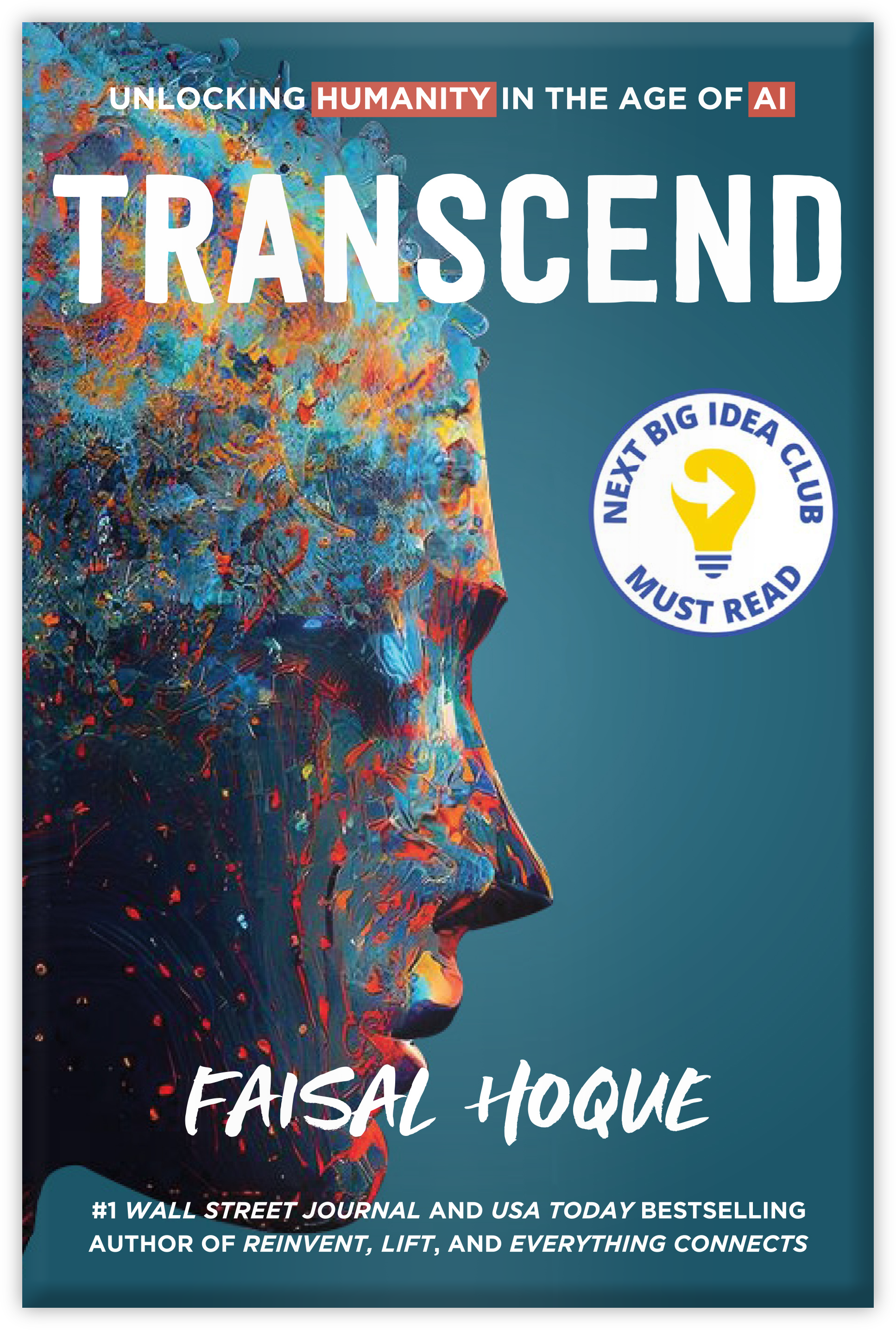
The key point is that LLMs are just one, highly visible, form of AI. Their distinctive feature in relation to other types of AI is that they are able to “understand” and generate text like human beings. That’s why we can talk to LLM-powered chatbots without any prior knowledge or training beyond knowing a language. LLMs are a profound development in AI, and it is perfectly reasonable to be excited by them. But there are many other different applications of AI, and many of these are also potentially revolutionary. When we consider how to use AI, then, we need to think beyond the potential of LLMs, even if these will be a critical source of easily accessible AI-powered tools for most of us.
The hype around AI comes in two flavors — positive and negative. The positive hype says that AI will make everything better. The negative hype says that it will literally destroy us. Having looked at the capabilities of foundation models and generative AI, and how these come together in LLMs, we are in a good position to understand the positive hype. But what about the negative side? What explains the worries?
One reason that many serious people fear the trajectory AI is taking is the worry that AI will simply become too intelligent. And one typical way of analyzing this possibility is to talk in terms of AI capability. At present, we can usefully divide the potential capabilities of AI models into three main categories:
- Narrow AI (Weak AI). This type of AI is “narrow” in the sense that it has been trained to carry out certain predefined tasks and has little or no capability beyond that narrow range. For example, certain AI systems used in finance are trained to detect irregularities in credit card usage in order to flag potentially fraudulent transactions. This is an important capability but it is a narrowly focused one.
- Artificial General Intelligence (AGI; Strong AI). When we try to learn new things, we typically rely on skills and knowledge that we already possess. For example, when we learn a new language, we rely on our skills of reading, writing, speaking, and listening. This is a remarkable feature of human intelligence — once we have a set of tools, it opens up virtually unlimited possibilities for learning. This type of intelligence can be called “general” because it is flexible and capable of adapting to new tasks and contexts. We can think of it as being something like a meta-capability: the capability of acquiring new capabilities at will. AI that has similar capabilities to humans in this regard can be called General or Strong AI.
- Super AI (Artificial Superintelligence). Super AI is like AGI, in the sense that its ability to perform tasks is not constrained by context-specific programming but differs in the scale of its power. Where AGI operates at a level analogous to that of a human, Super AI has the potential to be vastly more intelligent than any human being, with capabilities that scale with available processing power. It also has the capacity to act as an autonomous agent — to have its own desires, emotions, needs, and beliefs.
Now, before we can move on to using these levels of capability to explain some of the major fears around the development of AI, it is worth pointing out that this taxonomy is also useful in other ways. It helps us think about the different features that AI systems might have or lack, and it also provides a handy framework for keeping track of AI development. For example, when confronted with the latest AI hype story, we can ask: Is this a development toward AGI? If it isn’t, then is it a development within narrow AI? Is it doing something that other examples of narrow AI do but better or cheaper? Or is it a new application of existing AI capability?
This categorization also allows us to see that there is a big difference between the development of AI per se and its usefulness to any given person or organization. In terms of AI development as such, a new application of existing AI capability is not very exciting — it’s just more of the same. For an individual or an organization, however, a new application could be nothing short of revolutionary. An AI that specializes in analyzing legal documents might be just another boring iteration of the same fundamental technology to an AI researcher, but to a paralegal or an attorney, it could mark the end of their career or the beginning of a newly empowered phase in their life. Which is to say that the technical advance might be negligible while the practical impact is potentially life-changing.
[Source Photo: zapp2photo / Adobe Stock]
Original article @ BIG THINK.